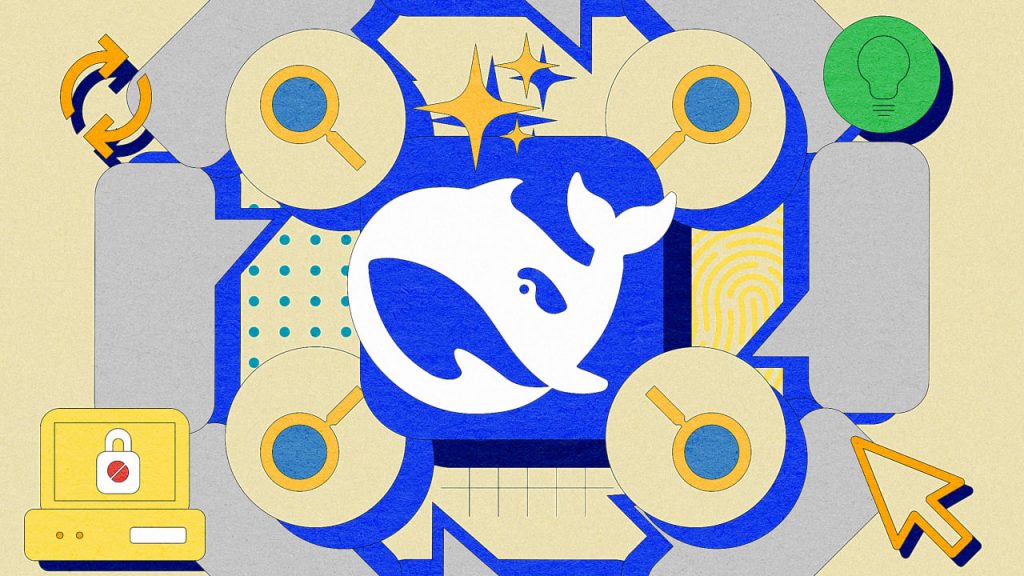
Twenty-four hours before the White House and Silicon Valley announced the $500 billion Project Stargate to secure the future of AI, China dropped a technological love bomb called DeepSeek.
DeepSeek R1 is a whole lot like OpenAI’s top-tier reasoning model, o1. It offers state-of-the art artificial thinking: the sort of logic that doesn’t just converse convincingly, but can code apps, calculate equations, and think through a problem more like a human. DeepSeek largely matches o1’s performance, but it runs at a mere 3% the cost, is open source, can be installed on a company’s own servers, and allows researchers, engineers, and app developers a look inside and even tune the black box of advanced AI.
In the two weeks since it launched, the AI industry has been supercharged with fresh energy around the products that could be built next. Through a dozen conversations with product developers, entrepreneurs, and AI server companies, it’s clear that the worried narratives most of us have heard about DeepSeek—it’s Chinese propaganda, it’s techie hype—doesn’t really matter to a free market.
“Everyone wants OpenAI-like quality for less money,” says Andrew Feldman, CEO and cofounder of the AI cloud hosting service Cerebras Systems that is hosting DeepSeek on its servers. DeepSeek has already driven down OpenAI’s own pricing on a comparable model by 13.6x. Beyond cost, DeepSeek is also demonstrating the values of open technologies versus closed, and wooing interest from Fortune 500s and startups alike. OpenAI declined an interview for this piece.
“Not to overstate it, but we’ve been in straight up giddy mode over here since [DeepSeek] came out,” says Dmitry Shevelenko, chief business officer at Perplexity, which integrated DeepSeek into its search engine within a week of its release. “We could not have planned for this. We had the general belief this is the way the world could go. But when it actually starts happening you obviously get very excited.”
Looking back five years from now, DeepSeek may or may not still be a significant player in AI, but its arrival will be considered a significant chapter in accelerating our era of AI development.
The new era of low-cost thought, powered by interoperability
Krea—an AI-based creative suite—had long considered adding a chatbot to the heart of its generative design tools. When DeepSeek arrived, their decision was made. Krea spent 72 hours from the time R1 was announced to integrating it as a chat-based system to control their entire editing suite.
Released on a Monday, the team realized by that afternoon that DeepSeek’s APIs worked with their existing tools, and it could even be hosted on their own machines. By Tuesday, they were developing a prototype, coding and designing the front end at the same time. By 3 a.m. Wednesday, they were done, so they recorded a demo video and shipped it by 7 a.m.
“That’s part of our culture; every Wednesday we ship something and do whatever it takes to get it done,” says cofounder Victor Perez. “But it’s a type of marketing that’s actually usable. People want to play with DeepSeek, and now they can do it with Krea.”
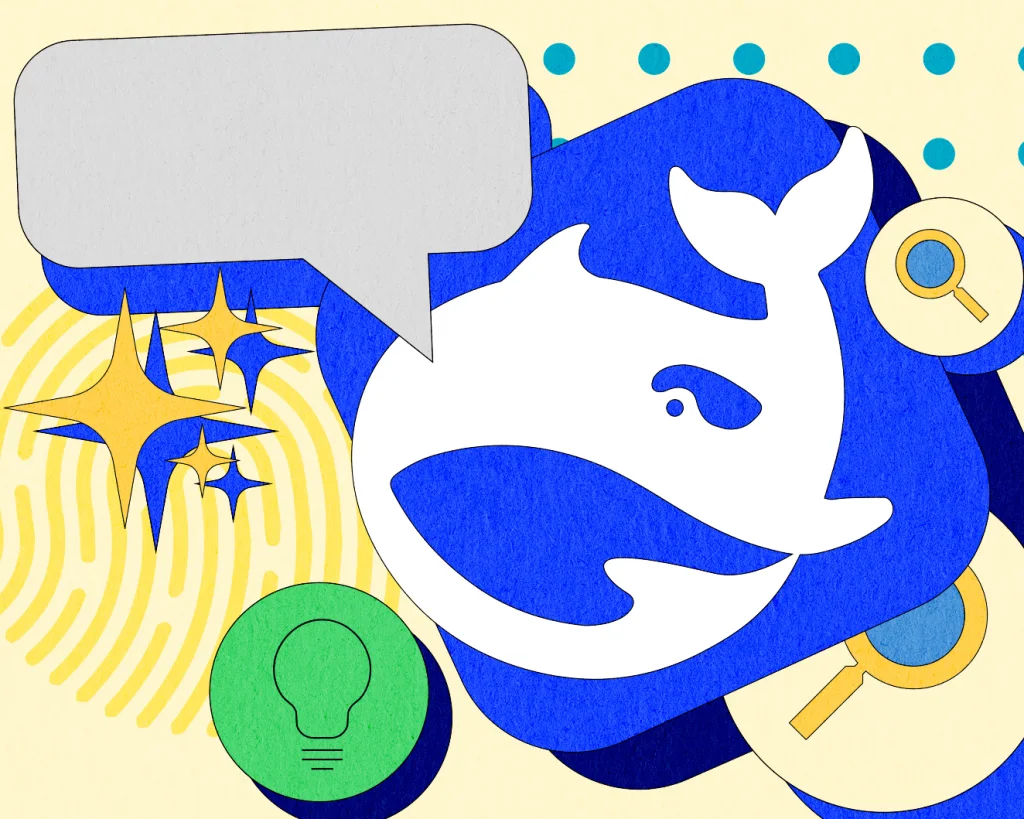
Krea’s story illustrates how fast AI is moving, and how product development in the space largely hinges on whatever model can deliver on speed, accuracy, and cost. It’s the sort of supply-meets-demand moment that’s only possible because of a shift underway in AI development. The apps we know are increasingly powered by AI engines. But something most people don’t realize about swapping in and out a large language model like R1 for 03, or ChatGPT for Claude, is that it’s remarkably easy on the backend.
“It would literally be a one-line change for us,” says Sam Whitmore, cofounder of New Computer. “We could switch from o3 to DeepSeek in like, five minutes. Not even a day. Like, it’s one line of code.”
A developer only needs to point a URL from one AI host to another, and more often than not, they’re discovering the rest just works. The “prompts” connecting software to AI engines still return good, reliable answers. This is a phenomenon we predicted two years ago with the rise of ChatGPT, but even Perez admits his pleasant surprise.
“Developers of [all] the models are taking a lot of care for this integration to be smooth,” he says, and he credits OpenAI for setting API standards for LLMs that have been adopted by Anthropic, DeepSeek, and a host of others. “But the [AI] video and image space is still a fucking mess right now,” he laughs. “It’s a completely different situation.”
Why DeepSeek is so appealing to developers
In its simplest distillation, DeepSeek R1 gives the world access to AI’s top tier thinking machine, which can be installed and tuned on local computers or cloud servers rather than connecting to OpenAI’s models hosted by Microsoft. That means developers can touch and see inside the code, run it at a fixed cost on their own machines, and have more control over the data.
Called inference models, this generation of reasoning AI works differently than the large language models like ChatGPT. When presented with a question, they follow several logical paths of thought to attempt to answer it. That means they run far slower than your typical LLM, but for heavy reasoning tasks, that time is the expense of thinking.
Developing these systems is computationally incredible. Even before the advanced programming methods were involved, DeepSeek’s creators fed the model 14.8 trillion pieces of information known as “tokens,” “which constitute a significant portion of the entire internet,” notes Iker García-Ferrero, a machine researcher at Krea. From there, reasoning models are trained with psychological rewards. They’re asked a simple math problem. The machine guesses answers. The closer it gets to right, the bigger the treat. Repeat countless times, and it “learns” math. R1 and its peers also have an additional step known as “instructional tuning,” which requires all sorts of hand-made examples to demonstrate, say, a good summary of a full article, and make the system something you can talk to.
“Some of their optimizations have been overhyped by the general public, as many were
already well known and used by other labs,” concedes García-Ferrero, who notes the biggest technological breakthrough was actually in an R1 “zero” sub model few people in the public are talking about because it was built without any instructional tuning (or expensive human intervention).
But the reason R1 took off with developers was the sheer accessibility of high tech AI.
“[Before R1], there weren’t good reasoning models in the open source community,” says Feldman, whose company Cerebras has constructed the world’s largest AI processing chip. “They built upon open research, which is what you’d want from a community, and they put out a comprehensive—or a fairly comprehensive paper on what they did and how.”
A few beats later, Feldman echoes doubt shared by many of his peers. “[The paper] included some things that are clearly bullshit . . . they clearly used more compute [to train the model] than they said.” Others have speculated R1 may have queried OpenAI’s models to generate otherwise expensive data for its instructional tuning steps, or queried o1 in such a way that they could deconstruct some of the black box logic at play. But this is just good old reverse engineering, in Feldman’s eyes.
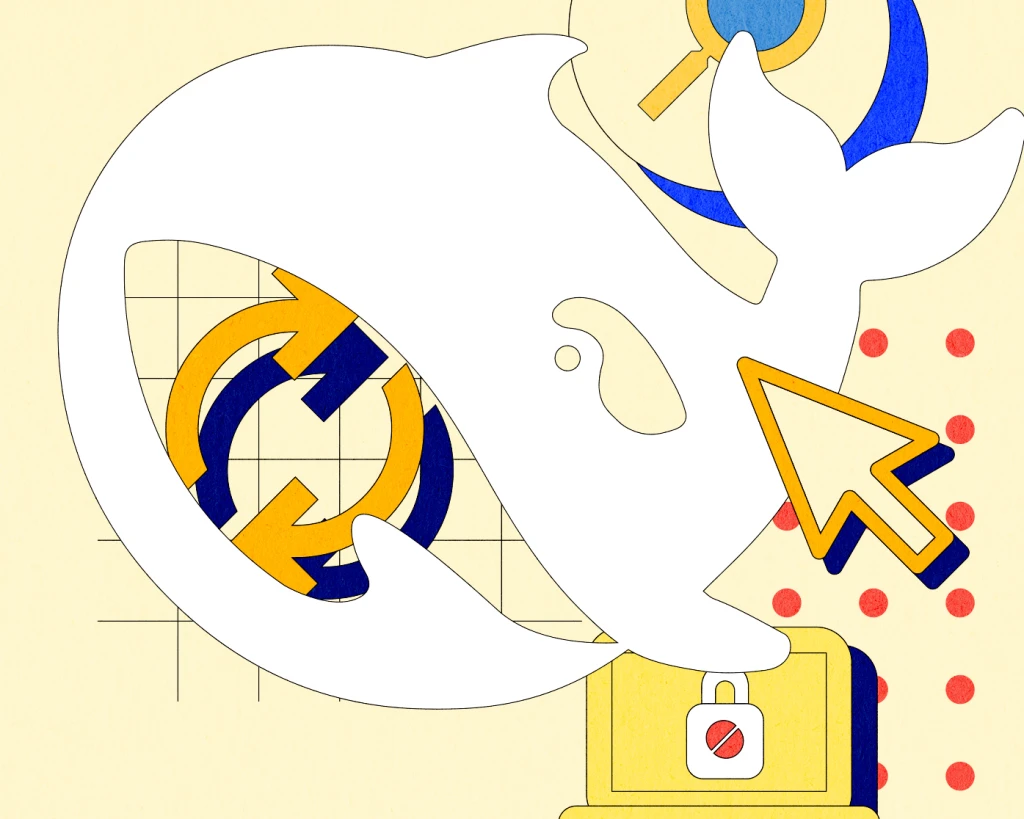
“If you’re a car maker, you buy the competitor’s car, and you go, ‘Whoa, that’s a smooth ride. How’d they do that? Oh, a very interesting new type of shock!’ Yeah, that’s what [DeepSeek] did, for sure.”
China has been demonized for undercutting U.S. AI investment with a free DeepSeek, but it’s easy to forget that, two years ago, Meta did much the same thing when, trailing Microsoft and Google in the generative AI race, it released LLaMa as the first open source AI of early LLMs. There was one difference, however: The devil is in the details with open source agreements, and while LLaMa still includes provisions stopping its commercial use by Meta’s competitive companies, DeepSeek used MIT’s gold standard license that blows it wide open for anything.
Now that R1 is trained and in the wild, the how, what, and why matter mostly to politicians, investors, and researchers. It’s a moot point to most developers building products that leverage AI engines.
“I mean, it’s cool,” says Jason Yuan, cofounder of the AI startup New Computer. “We’re painters, and everyone’s competing over giving you better and cheaper paints.”
A wave of demand for DeepSeek
Feldman describes the last two weeks at Cerebras as “overwhelming,” as engineers have been getting R1 running on their servers to feed clients looking for cheap, smart compute.
“It’s like, every venture capitalist calls you and says, ‘I got a company that can’t find supply. Can you help out?’ I’m getting those three, four times a day,” says Feldman. “It means you’re getting hundreds of requests through your website. Your sales guys can’t return calls fast enough. That’s what it’s like.”
These sentiments are shared by Lin Qiao, CEO and cofounder of the cloud computing company Fireworks, which was the first U.S.-based company to host DeepSeek R1. Fireworks has seen a 4x increase in user signups month-over-month, which it attributes to offering the model.
Qiao agrees that part of the appeal is price. I’ve heard estimates that R1 is about 3% the cost of o1 to run, and Qiao notes that on Fireworks, they’re tracking it as “5X cheaper than o1.”
Notably, OpenAI responded to DeepSeek with a new model released last week called o3 mini. According to Greg Kamradt, the founder of ARC Prize, a nonprofit AI benchmarking competition, o3 mini is 13.6x cheaper than o1 processing tasks. Cerebras admits o3 is all around more advanced than DeepSeek’s R1, but claims the pricing is comparable. Fireworks contends o3 mini is still less expensive to query than R1. The truth is that costs are moving targets, but the bigger takeaway should be that R1 and o3 mini are similarly cheap. And developers don’t need to bet on either horse today to take advantage of the new competition.
“Our philosophy is always to try all models,” writes Ivan Zhao, founder and CEO of Notion, over email. “We have a robust eval system in place, so it’s pretty easy to see how each model performs. And if it does well, is cost effective, and meets our security and privacy standards, then we’ll consider it.”
DeepSeek offers transparent thought for the first time
Shevelenko insists that integrating DeepSeek into Perplexity was more than a trivial effort. “I wouldn’t put it in the mindless bucket,” he says. But the work was still completed within a week. In many ways, the larger concern for integration was not, would it function, but could Perplexity mitigate R1’s censorship on some topics as it leveraged AI for real time internet queries.
“The real work was we quickly hired a consultant that’s an expert in Chinese censorship and misinformation, and we wanted to identify all the areas in which the DeepSeek model was potentially being censored or propagating propaganda,” says Shevelenko. “And we did a lot of post-training in a quick time frame . . . to ensure that we were answering any question neutrally.”
But that work was worth it because, “it just makes Perplexity better,” he says.
Shevelenko is not talking in platitudes; with DeepSeek, Perplexity can do something the world has never seen before: Offer a peek inside how these AIs are actually thinking through a problem. This feature of R1 is called “chain-of-thought.”
Perplexity always offered some transparency in its front end, listing the websites it was crawling on your behalf to answer a question. But now, it will list the prompts as R1 literally talks to itself, step by step, as it reasons through an answer.
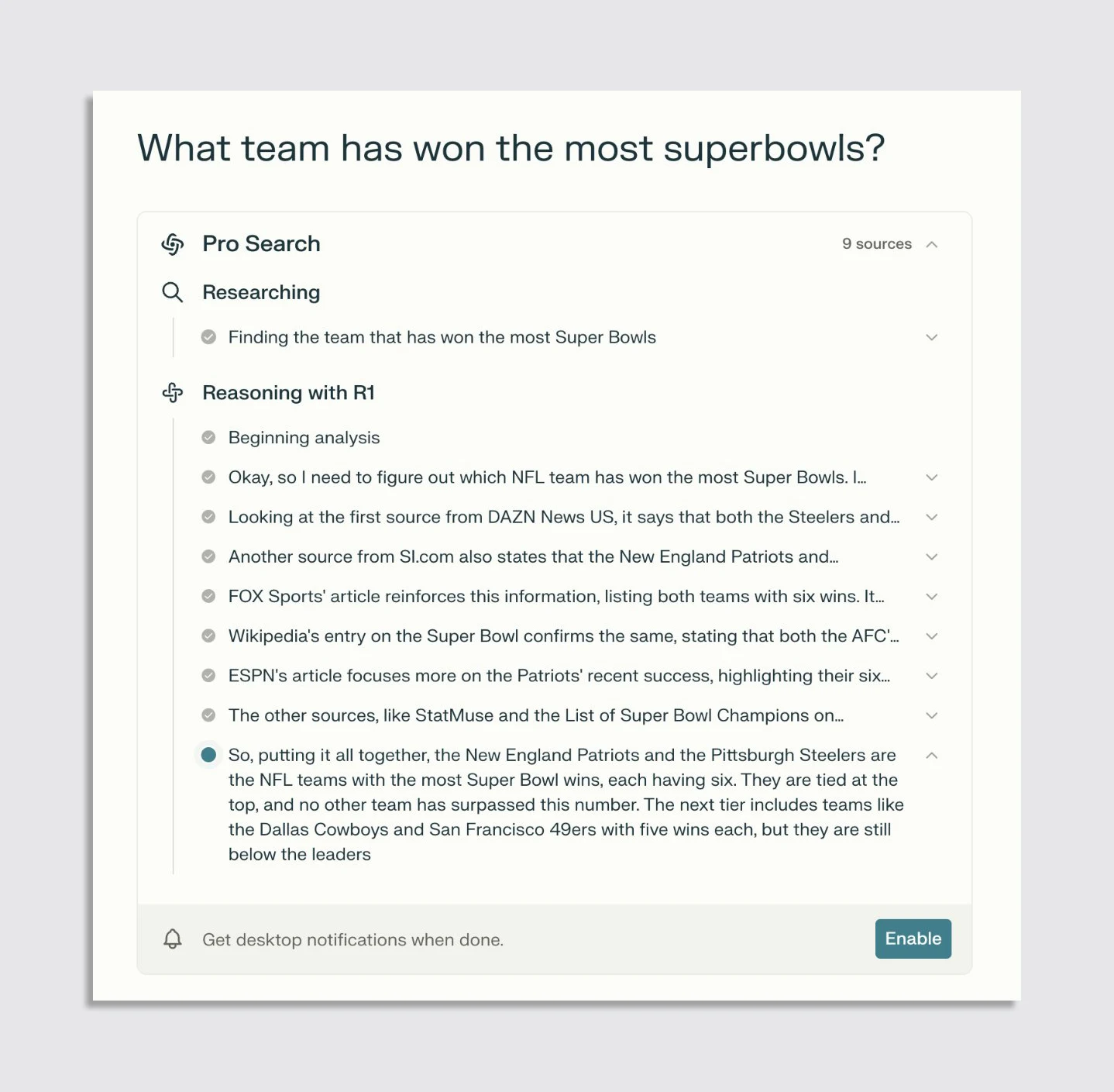
“OpenAI, for competitive purposes, never exposed [chain-of-thought]. One of Perplexity’s strengths is UI; we are able to quickly figure out an elegant way of showing you how the model is thinking in real time,” says Shevelenko. “There’s a curiosity and a utility to it. You can see where the thinking may have gone wrong and reprompt, but more than anything, part of the whole product law at Perplexity is not that you always get the best answer in one shot, it’s that you’re guided on the way to ask better and better questions. It makes you think of other questions.”
Seeing AI reasoning laid bare also creates more intimacy with the user. “The biggest problem of AI right now, how can we trust it? Because we all know AI can hallucinate,” says Qiao. However, if transparent thought can bridge this gap of trust, then she imagines developers will begin to do a lot more we can’t think of yet with all of this thinking data.
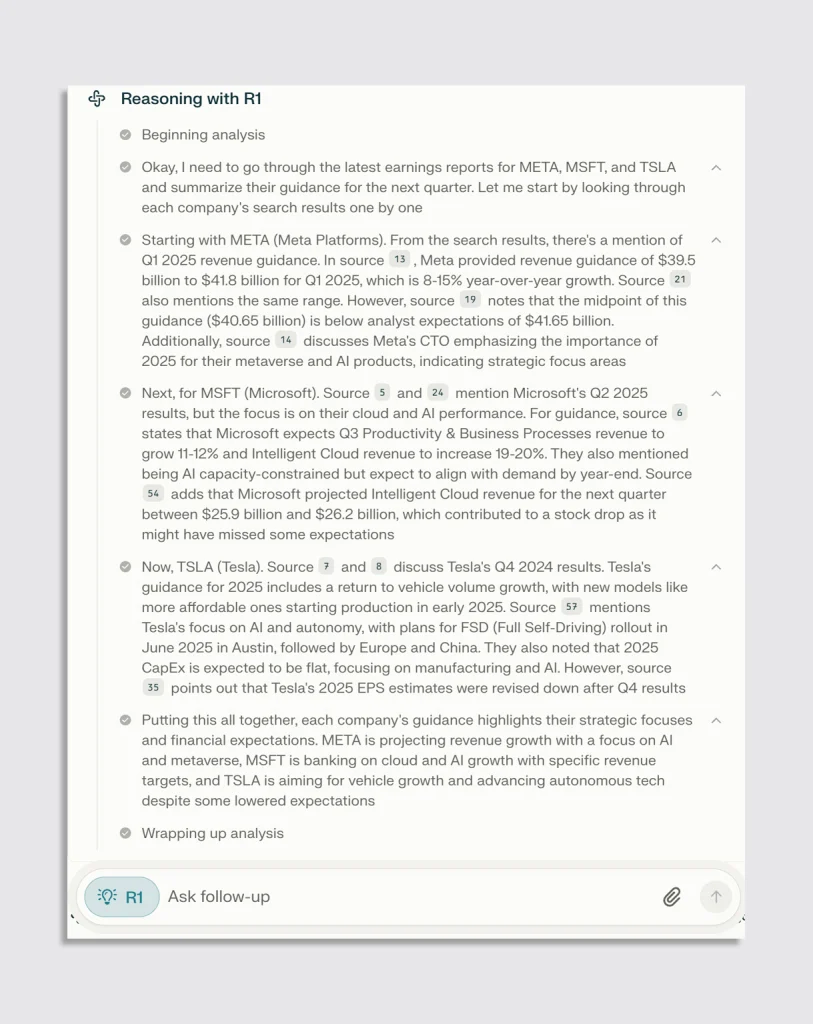
“There may be products built directly on top of chain-of-thought. Those products could be general search, or all kinds of assistants: coding assistant, teaching assistant, medical assistants.” She also believes that, while AI has been obsessed with the assistant metaphor since the launch of ChatGPT, transparent thought will actually give people more faith in automated AI systems because it will leave a trail that humans (or more machines!) can audit.
Buying breathing room for the future
Even as debates about Chinese vs U.S. innovation rage on, the biggest single impact that DeepSeek will have is giving developers more autonomy and capability. Some, like Anthropic CEO Dario Amodei, argue that we are simply witnessing the known pricing and capability curve of AI play out. Others recognize the kick in the ass that DeepSeek offered an industry hooked on fundraising and opaque profit margins.
“There’s no way OpenAI would have priced o3 as low as they had it not for R1,” says Shevelenko. “It’s a bit of a moving target, once you have an open source drop it dramatically curves down the pricing for closed models, too.”
While nothing is to say that OpenAI or Anthropic won’t release a far more cutting edge model tomorrow that puts these systems to shame, this moving target is providing confidence to developers, who now see a path toward realizing implementations they’d only fantasized about, especially now that they can dip their own fingers into advanced AI.
R1 on its own is still relatively slow for many tasks; a question might take 30 seconds or more to answer, as it has a habit for obsessively double checking its own thinking, perhaps even burning extra energy than it needs to in order to give you an answer. But since it’s open source, the community can “distill” R1—think of it like a low cost clone—to run faster and in lower power environments.
Indeed, developers are already doing this. Cerebras demonstrated a race between its own distilled version of R1 to code a chess game against an o3 mini. Cerberus completed the task in 1.2 seconds versus 22 seconds on o3. Efficiencies, fueled by both internal developers and the open source community, will only make R1 more appealing. (And force proprietary model developers to offer more for less.)
At Krea, the team is most excited about the same thing that’s exciting the big AI server companies: They can actually task an engineer to adjust the “weights” of this AI (essentially tuning its brain like a performance vehicle). This might allow them to run an R1 model on a single GPU themselves, sidestepping cloud compute altogether, and it can also let them mix homebuilt AI models with it.
Being able to run models locally on office workstations, or perhaps even distilling them to run right on someone’s phone, can do a lot to reduce the price of running an AI company.
Right now, developers of AI products are torn between short term optimizations and long-term bets. While they charge $10 to $30 a month, those subscriptions make for a bad business today that’s really betting on the future.
“It’s really hard for any of those apps to be profitable because of the cost of doing intelligent workflows per person. There’s always this calculus you’re doing where it’s like, ‘OK, I know that it’s going to be cheap, long, long term. But if I build the perfect architecture right now with as much compute as I need, then I may run out of money if a lot of people use it in a month,” says Whitmore. “So the pricing curve is difficult, even if you believe that long term, everything will be very cheap.”
What this post-DeepSeek era will unlock, Whitmore says, is more experimentation from developers to build free AI services because they can do complicated queries for relatively little money. And that trend should only continue.
“I mean, the price of compute over the past 50 years has [nosedived], and now you have 30 computers in your house. Each of your kids has toys with it. Your TVs have computers in them. Your dishwashers have computers in them. Your fridges probably have five. If you look around, you got one in your pocket,” says Feldman. “This is what happens when the price of compute drops: You buy a shitload of it.”
And what this will mean for the UX of AI will naturally change, too. While the way most of us use AI is still based in metaphors of conversation, when it can reason ahead faster than we can converse, the apps of tomorrow may feel quite different—even living steps ahead of where we imagine going next.
“As humans, even the smartest of us, take time to reason. And right now, we’re used to reasoning models taking a bit of time,” says Yuan of New Computer. “But swing your eyes just a few months or even a year, and imagine thinking takes one second or less, or even microseconds. I think that’s when you’ll start seeing the quote unquote ‘AI native interfaces beyond chat.’”
“I think it’s even hard to kind of imagine what those experiences will feel like, because you can’t really simulate it. Even with science fiction, there’s this idea that thinking takes time,” he continues. “And that’s really exciting. It feels like this will happen.”